Title
Manual Labeling
Company
eBay @ Payment & Risk
2023.09 - 2023.11
My Role
UX Research
UI Design
UX Design
I designed Manual Labeling feature from 0 to 1, from researching, identifying problems, to designing and handing off for implementation. Subsequent data showed that my design effectively helped the company achieve its business goals by reducing 29% of the average time of the manual data labeling process.
To comply with my non-disclosure agreement, I have omitted and obfuscated confidential information in this case study. All information in this case study is my own and does not necessarily reflect the views & facts of the company.
Context
The eBay Payment & Risk Team trains and uses AI models to prevent payment fraud and other actions that could result in financial loss. This process relies heavily on extensive manual data labeling. While some labeling jobs not involving sensitive data are outsourced, there is still an internal team dedicated to handling labeling jobs that involve sensitive data and require specific domain knowledge.
The Internal Manual Labeling Team (IML) at eBay consists of three distinct roles: Data Scientists, who create labeling jobs; Labeling Managers, who assign these jobs; and Data Labelers, who complete the jobs and then return the labeled data to the Data Scientists for further processing.
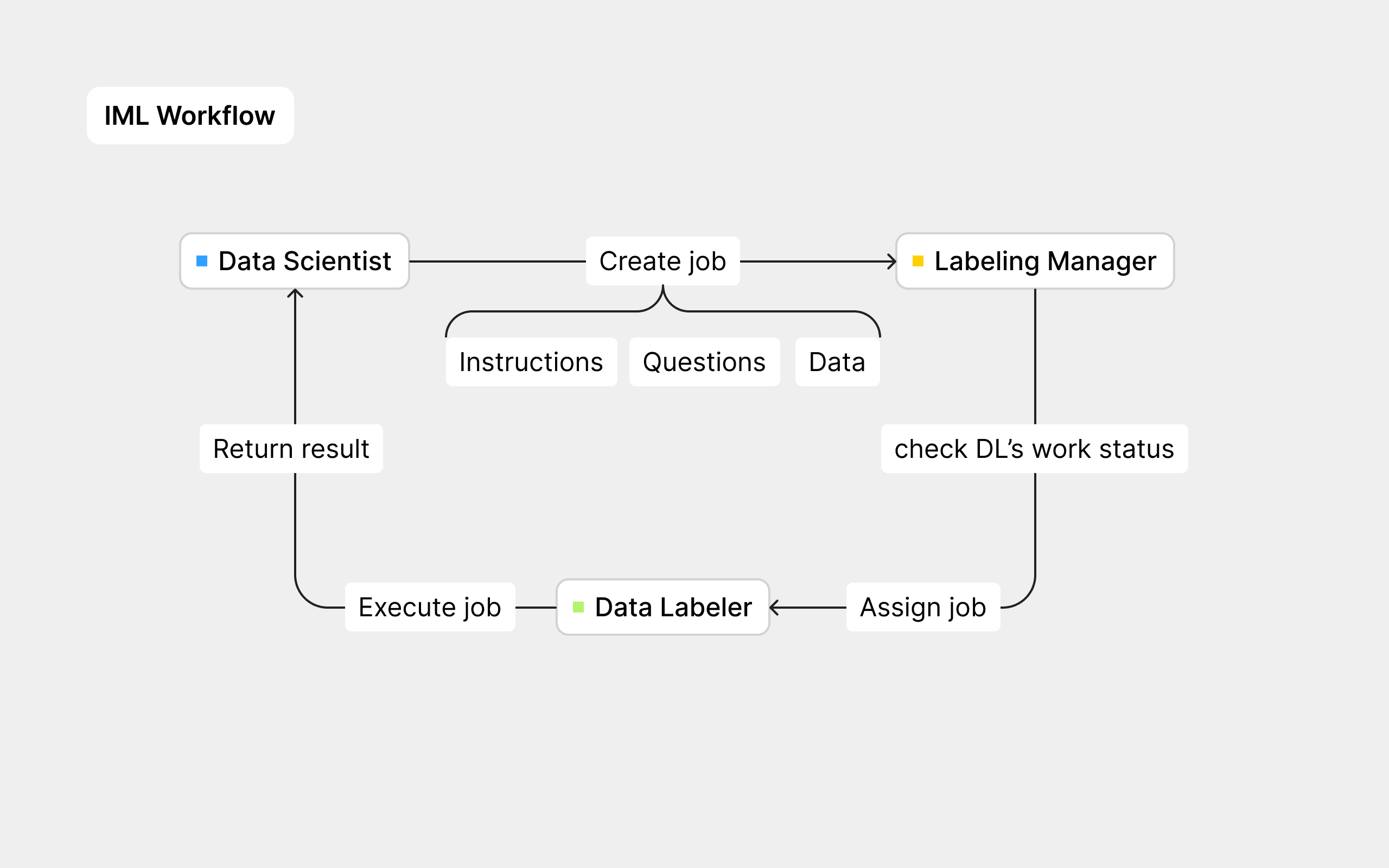
Business Problem & Objective
Due to the lack of specialized features in electronic spreadsheet tools like Google Sheets and Excel, tailored for labeling work, manual data labeling relying entirely on these tools is highly inefficient. This inefficiency results in excessively long completion times for labeling jobs, slowing down the update speed of AI models, affecting the accuracy of their detection capabilities, and increasing the risk of financial loss.
To improve efficiency without additional labor costs, we decided to develop an internal tool specifically for manual labeling. By doing so, we aim to reduce the labeling process time by an estimated 25%, meeting the needs of AI training and enhancing overall effectiveness.
Discovery
In the manual labeling process, each IML team role has specific tasks, and I aimed to design tailored workflows for each. To learn more about unmet user needs for each role, I planned different research methods for different goals, and then me & my PM worked together.
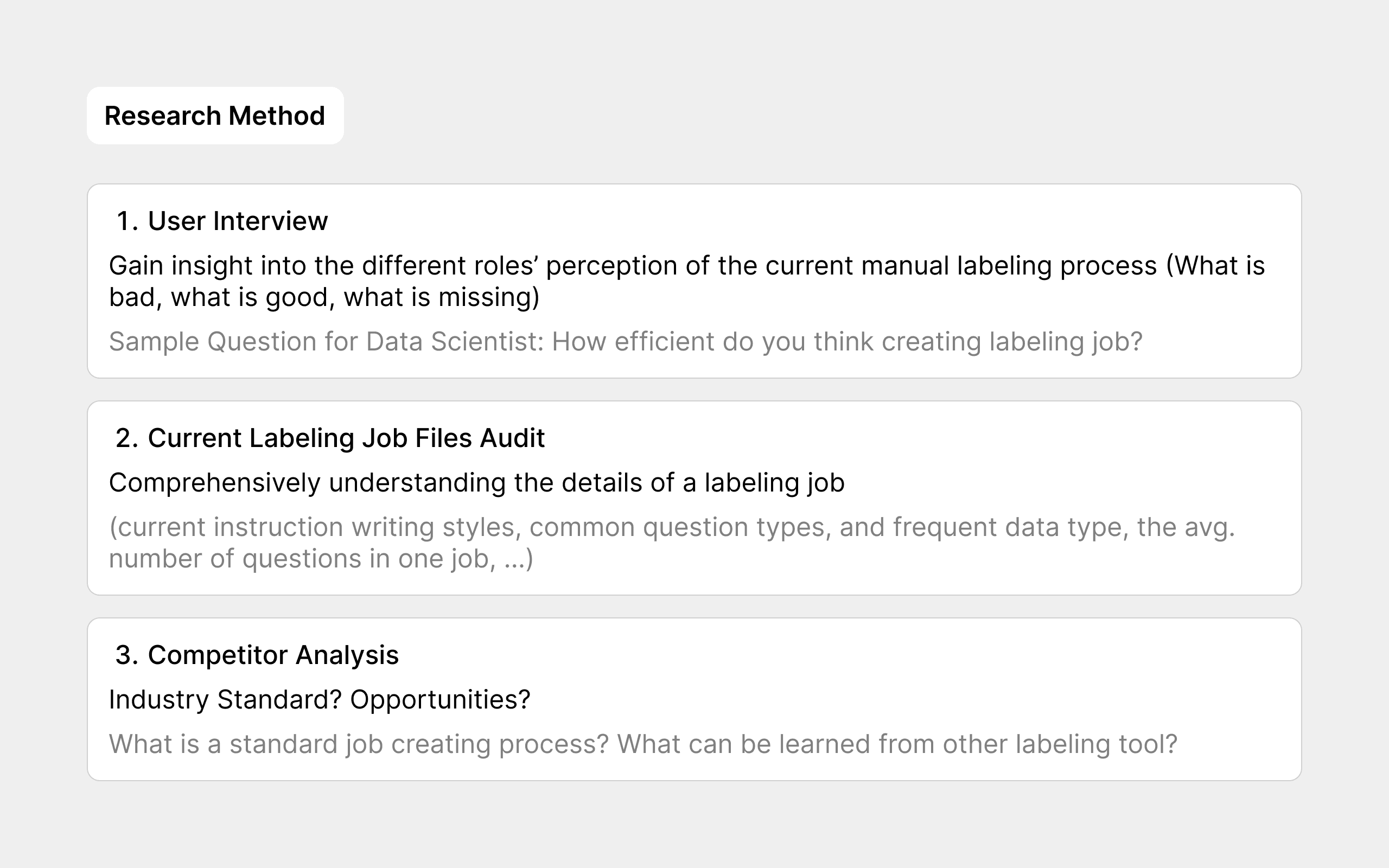
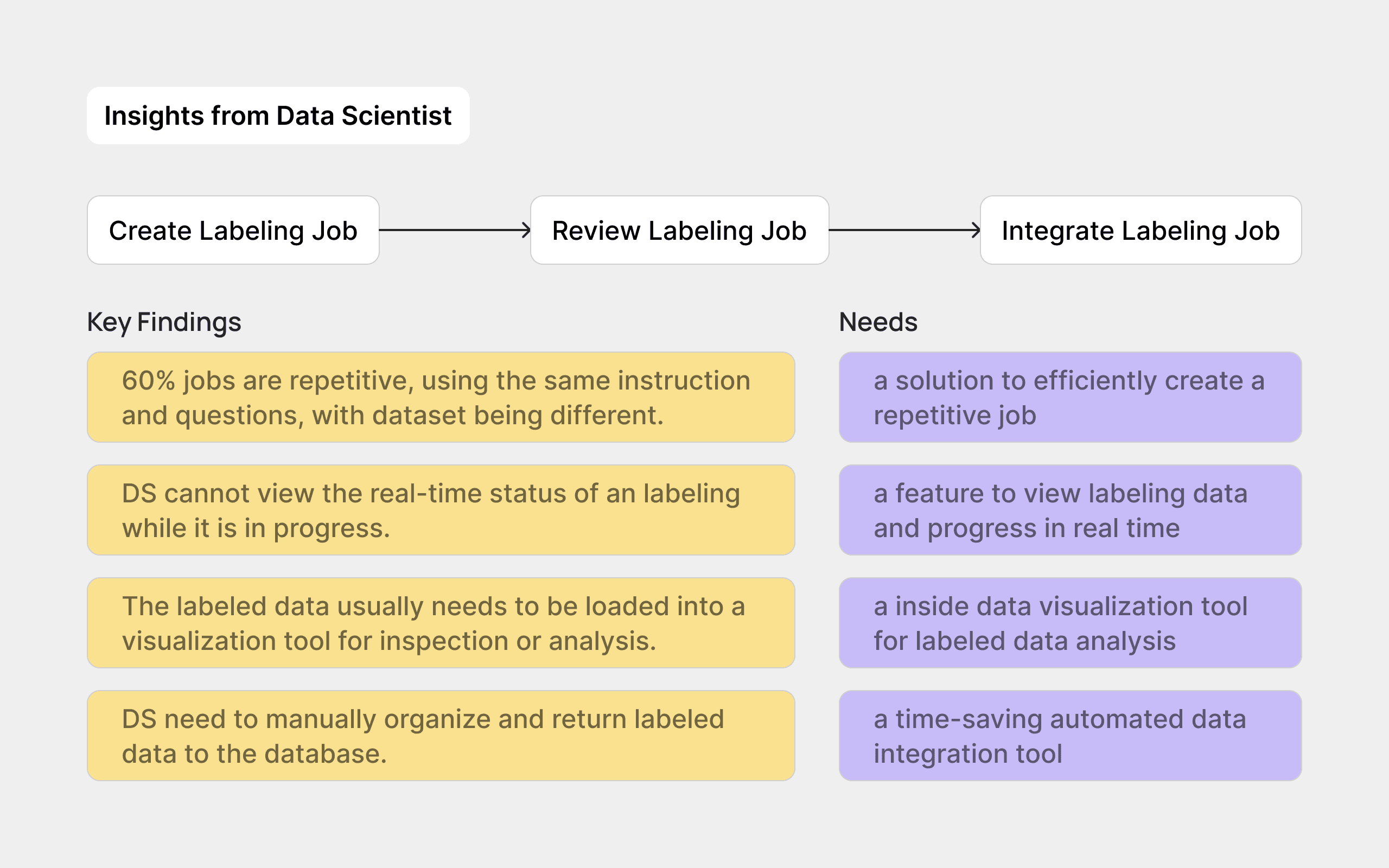
Findings & Needs from DS
Define
I established design goals for each role based on identified user needs in research and their proportion of work time. We aimed to satisfy specific requirements and expectations of different users while also achieving the broader objective of enhancing overall efficiency.
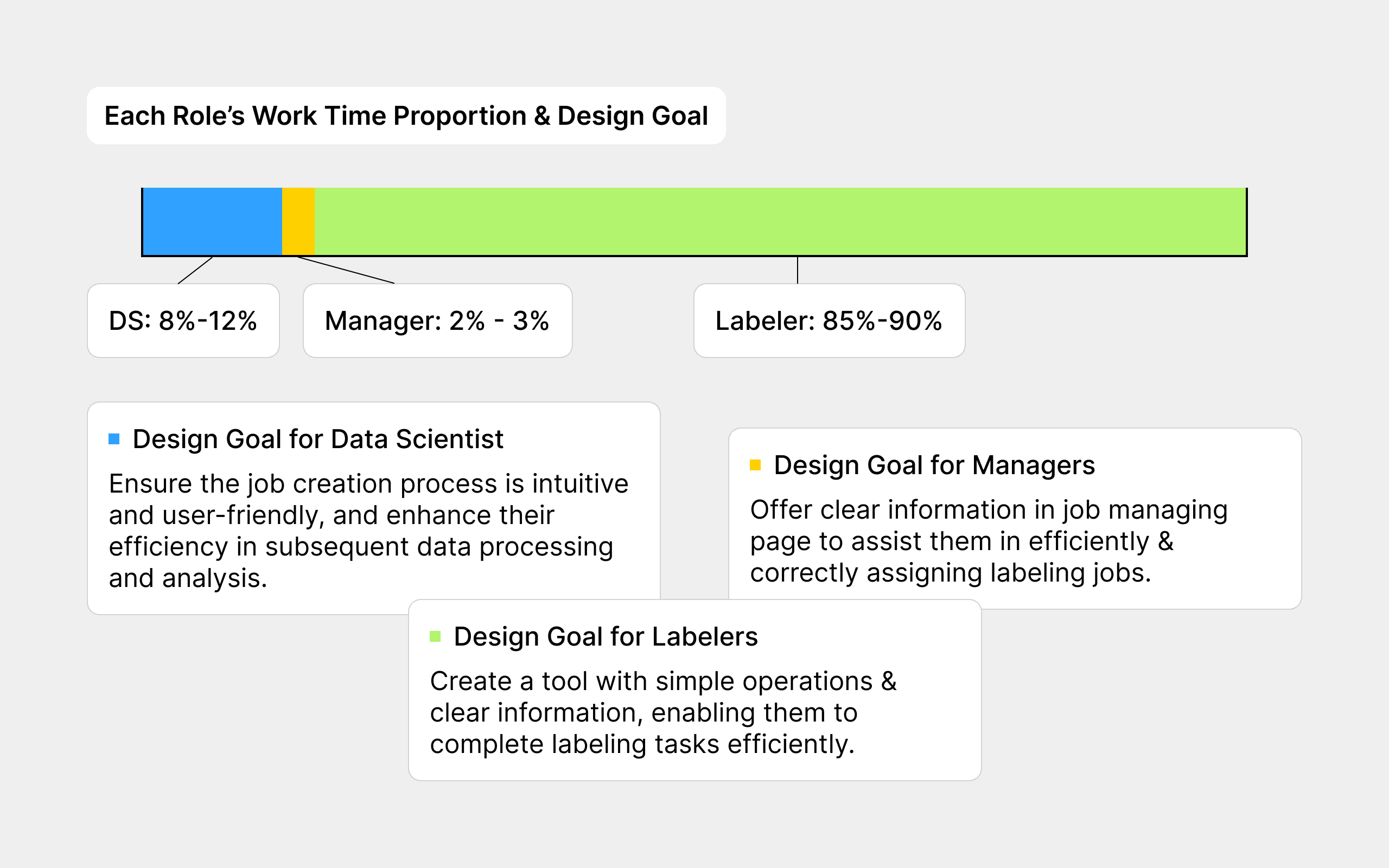
Design & Iteration
After defining our design goals for each role, I crafted wireframes to exploring the page structure, functionality, and user flow. Next, we quick tested these wireframes in team meetings, & rapidly iterated those wireframes for any identified design issues. I then promptly shifted to creating high-fidelity prototypes, as they offer a more precise representation of the product's final user experience.
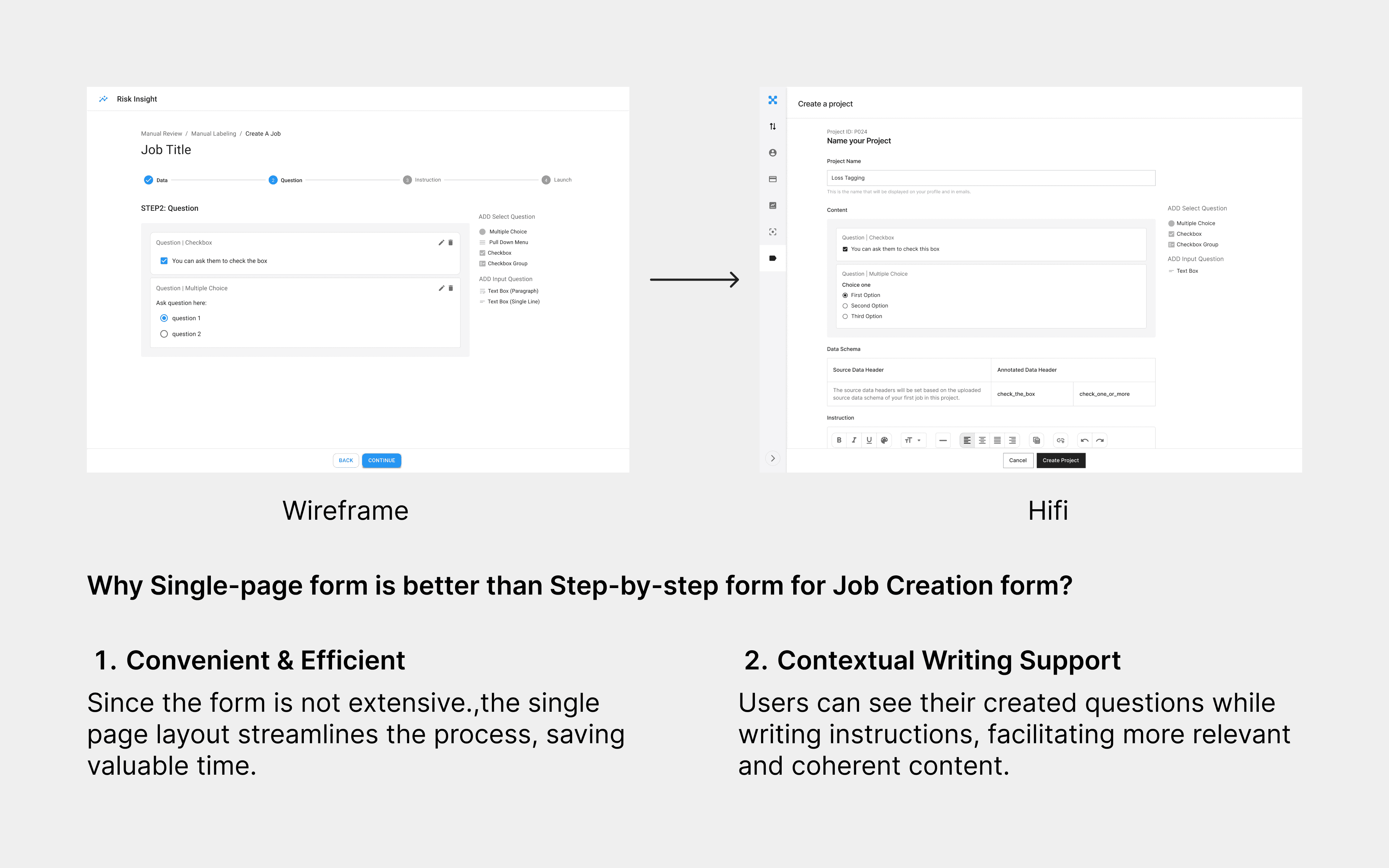
Iteration Ex.1: Step-by-step vs Single-page
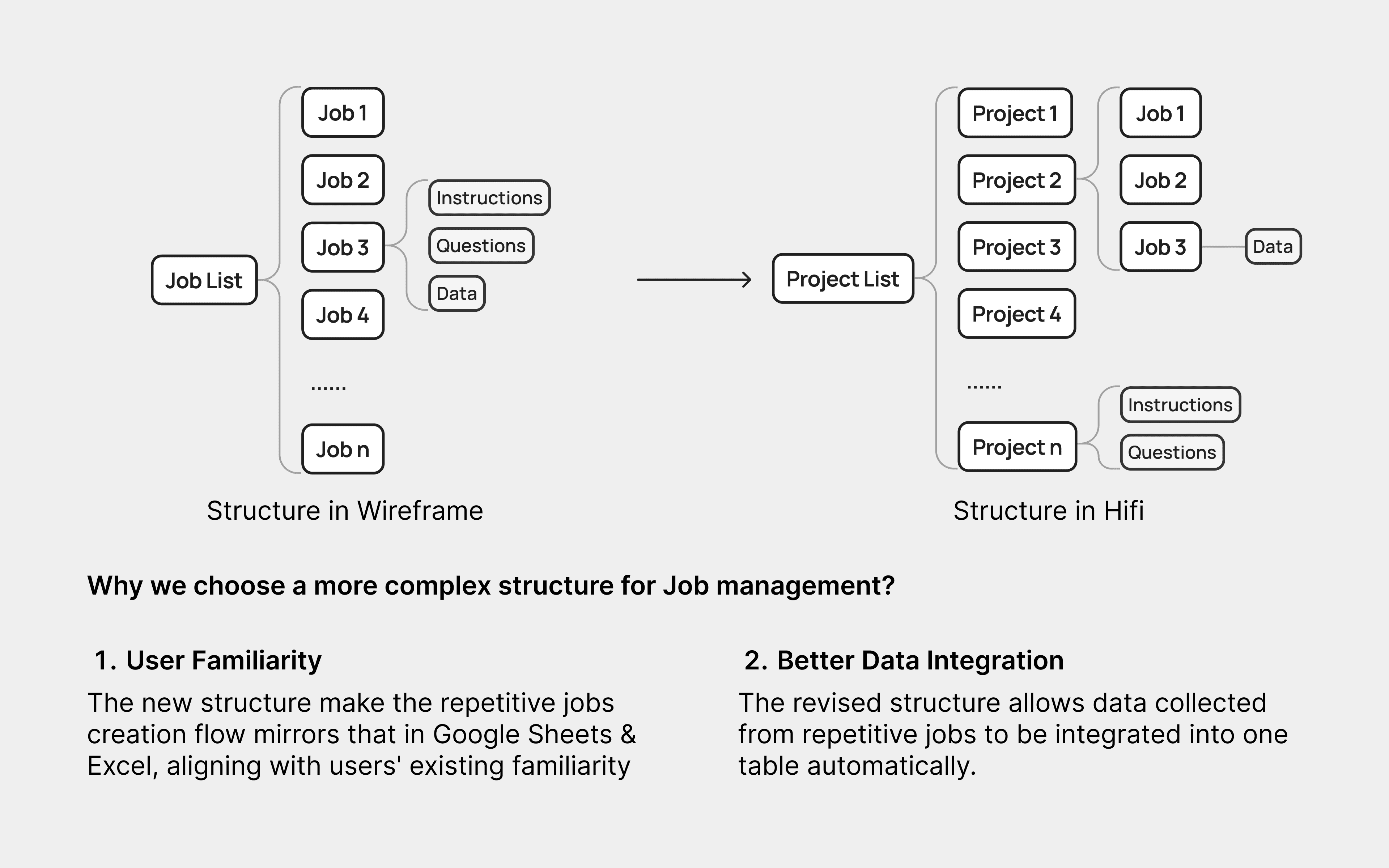
Iteration Ex.2: Why we choose a more complex structure?
To ensure that our product was both intuitive and efficient, I invited members from different roles to conduct two rounds of usability testing on the high-fidelity prototypes. The purpose of these tests was to validate the practicality and feasibility of the design. During the tests, all participants were assigned specific tasks, and by observing their interactions with the interface, we identified some issues that had previously gone unnoticed. Additionally, their feedback post-testing provided valuable insights for refining our solutions.
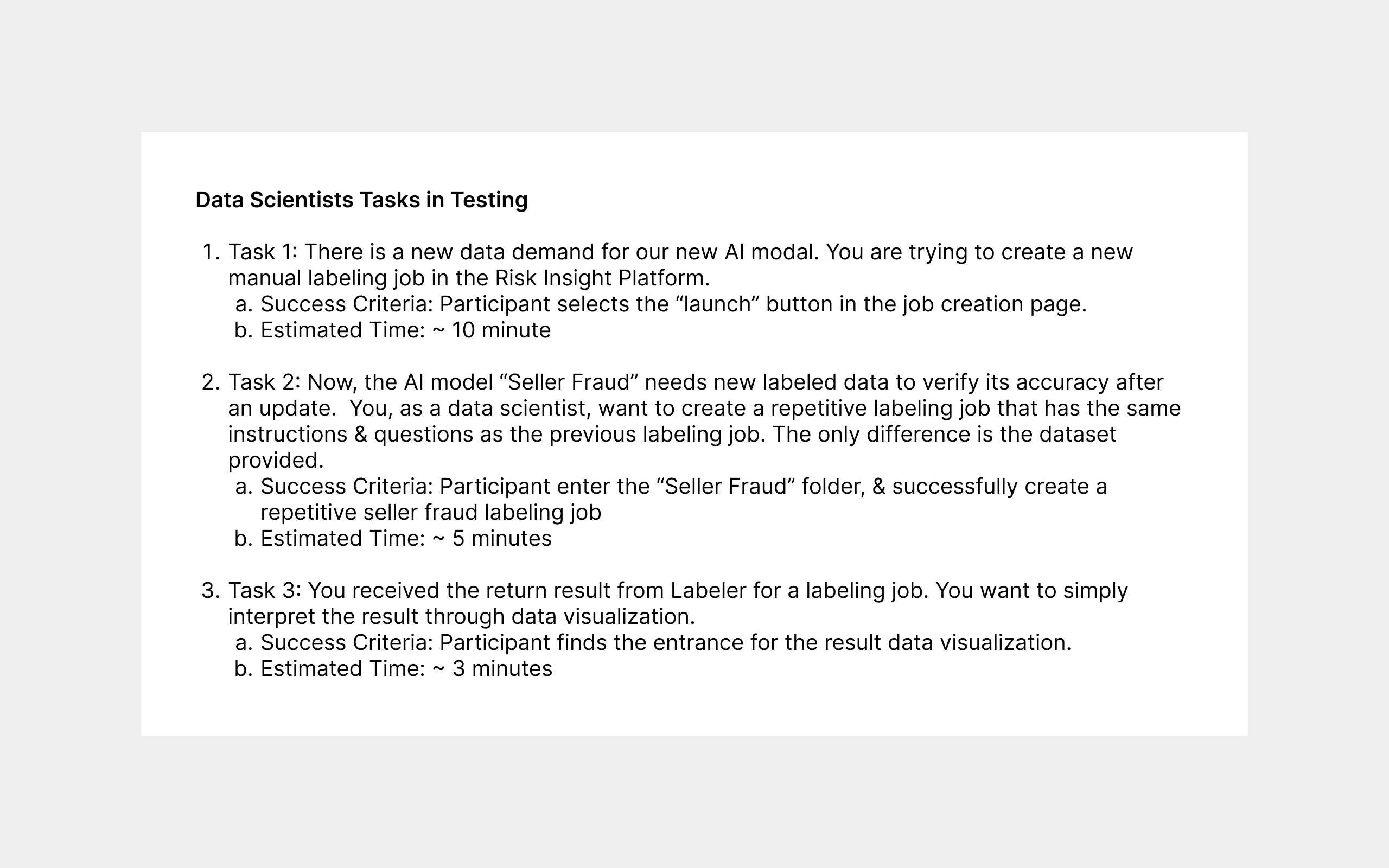
Tasks in Usability Testing Doc for Data Scientists
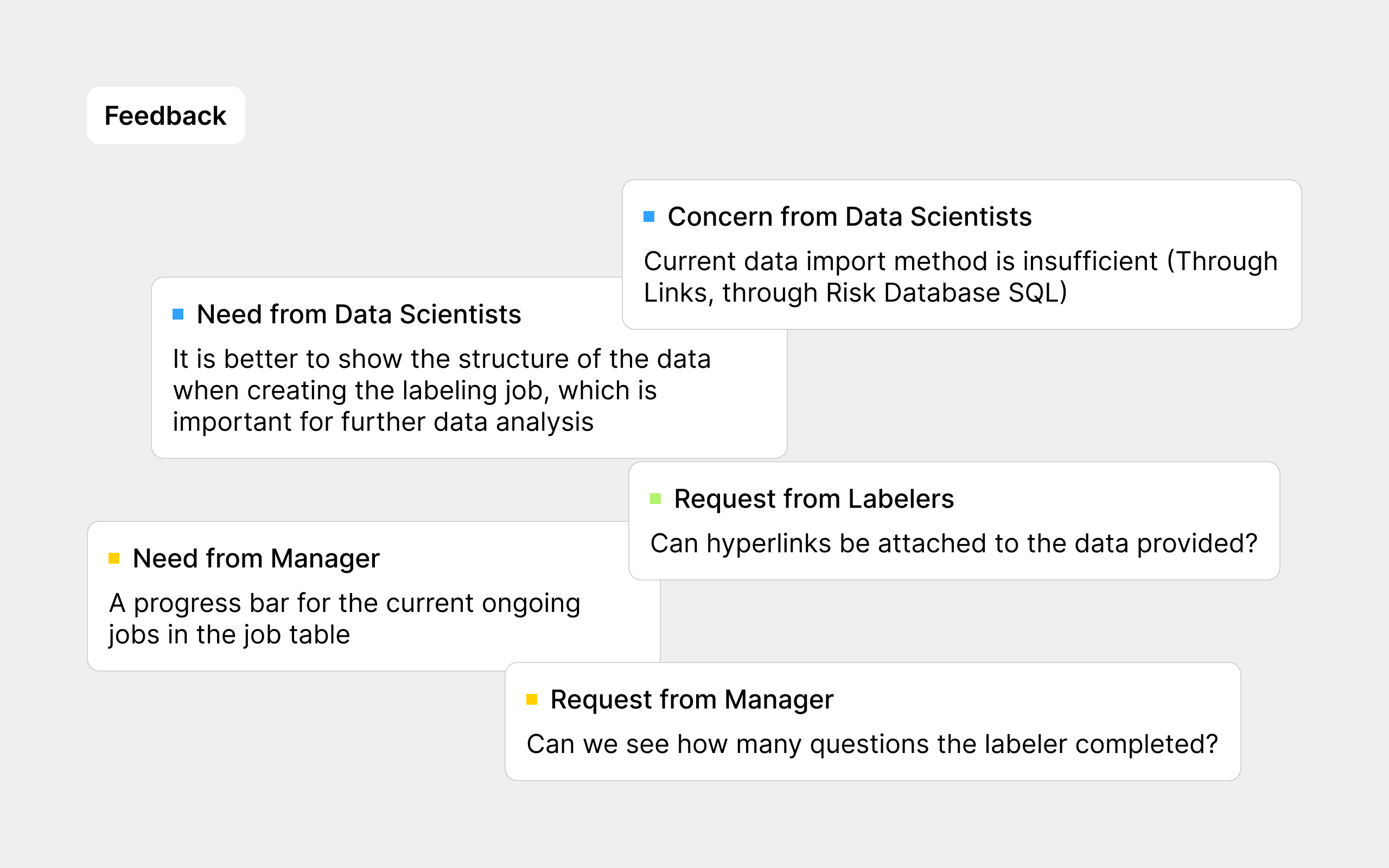
Feedback Example
Challenge
With only two and a half months for design and development, it was challenging to address all the needs identified in research and testing within this tight timeframe. To address this efficiently, I organized a collaborative workshop:
presented the pain points and insights I discovered to the team
discussed with the devs to understand the cost (in terms of workload) of solving each demand
prioritized features based on both user value and dev effort in MVP
Unselected feature is scheduled after the completion of MVP
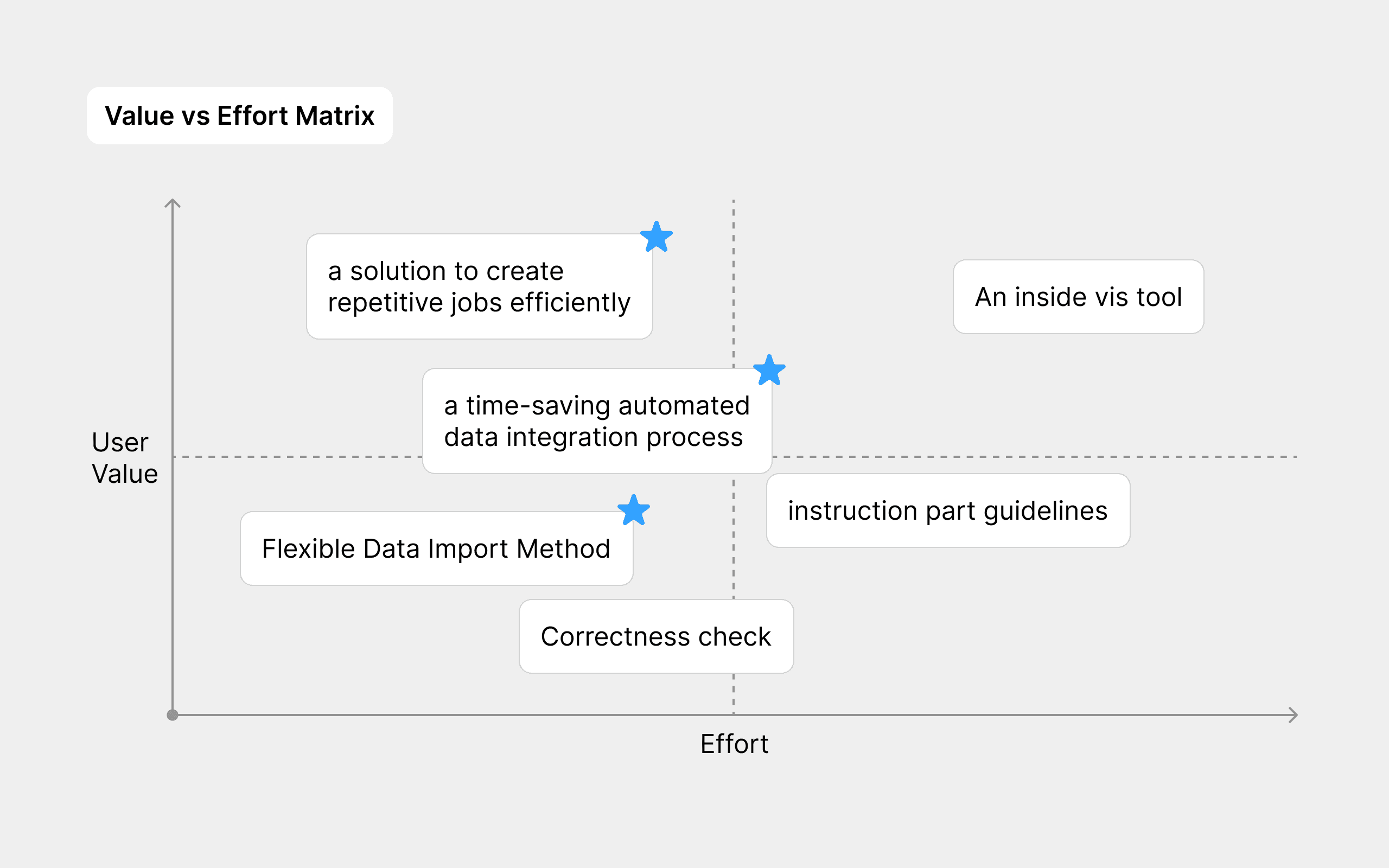
Result
After the new feature was launched, we conducted data tracking for three months. Thankfully, my colleagues still provided some data even after I left the company. By the second month, as the IML team became increasingly familiar with the new tool, the average time for data labeling was reduced by 23%. Impressively, by the third month, the average time for the labeling process decreased by 29%, exceeding our initial target. Furthermore, there is still significant potential for further efficiency improvements, as many features have yet to be added.
Data Scientist‘s Project Creation
Data Scientist‘s Job Creation
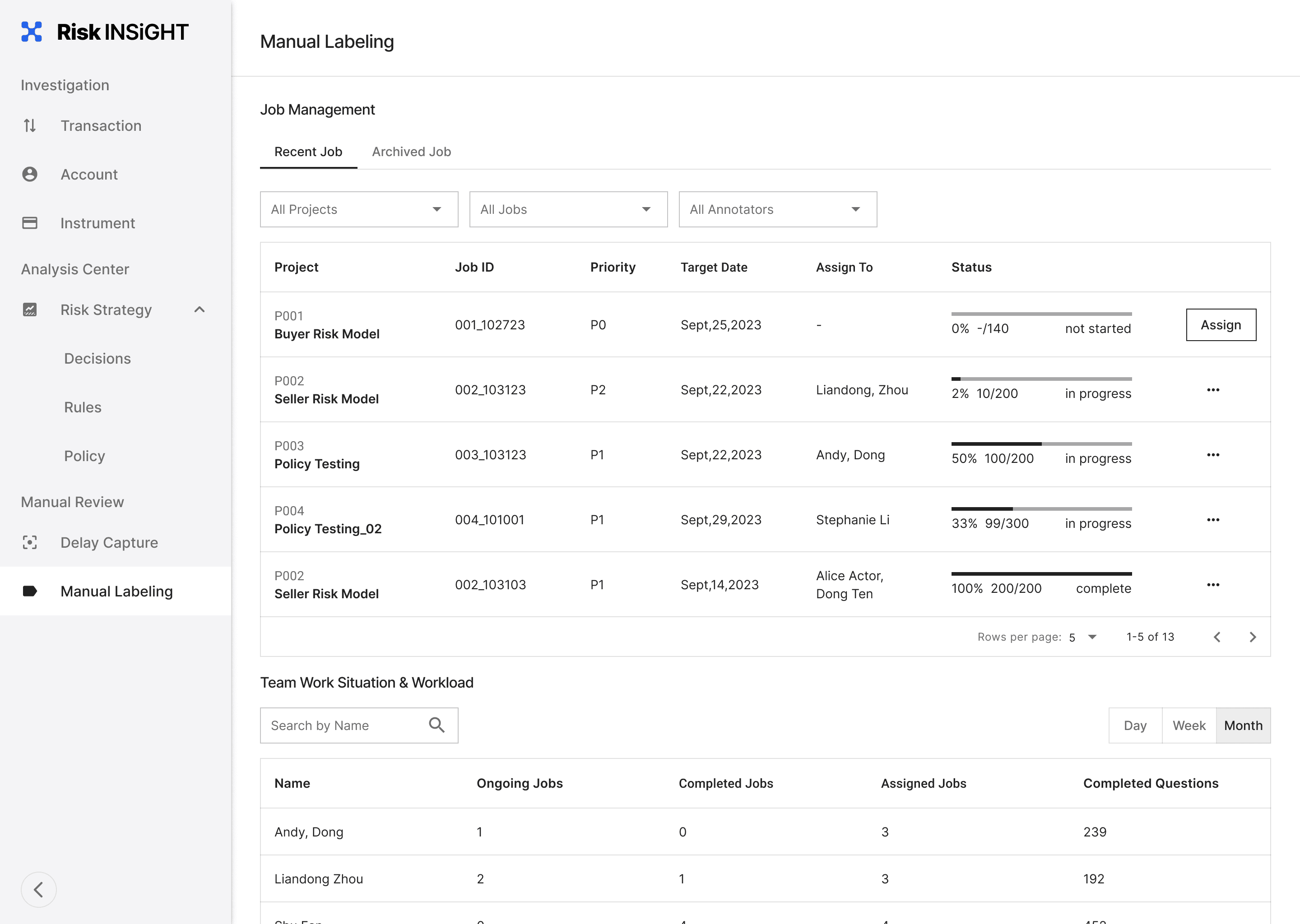
Labeling Manager's Job Management Tool
Data Labeler's Job Execution
Takeaways
I could sense that my efforts not only improved the overall process efficiency but also had a positive impact on their daily work. This was particularly true for the labelers, whose needs and experiences often went overlooked in the company due to various reasons. Before leaving the company, I received a message on Slack from one of the labelers:
"I love the new labeling feature!This is incredibly useful, I no longer have to deal with the cramped data on Google Sheets. Thank you, Lian."